Clin Res Cardiol (2023). https://doi.org/10.1007/s00392-023-02180-w
|
Impact of Effective Refractory Period Personalization on Prediction of Atrial Fibrillation Vulnerability
|
P. Martínez Díaz1, C. Götz1, A. Dasí I Martinez2, L. A. Unger3, A. Haas4, O. Dössel1, A. Luik4, A. Loewe1
|
1Institut für Biomedizinische Technik, Karlsruher Institut für Technologie (KIT), Karlsruhe; 2Department of Computer Science, University of Oxford, Oxford, UK; 3Klinik für Kardiologie und Angiologie, Universitätsklinikum Essen, Essen; 4Med. IV, Schwerpunkt Kardiologie, Angiologie und Internistische Intensivmedizin, Städtisches Klinikum Karlsruhe gGmbH, Karlsruhe;
|
Aim
Although the effective refractory period (ERP) is one of the main electrophysiological properties governing atrial tachycardia (AT) maintenance, ERP personalization is rarely taken into account when creating patient-specific computer models of the atria. State-of-the-art personalized models usually do not consider physiological ERP gradients but assume a homogeneous ERP distribution. Therefore, we evaluated the impact of incorporating clinical ERP measurements when creating in silico personalized models to predict vulnerability to atrial fibrillation (AF).
Methods
Clinical ERP measurements were obtained from two patients, each at 8 different locations in the atria. The pacing protocol for ERP identification consisted of trains of 7 S1 stimuli with a basic cycle length of 500ms followed by an S2 stimulus with a coupling interval between 300 and 200ms in decrements of 10ms until loss of capture. The atrial geometries acquired with the Rhythmia HDx 3D mapping system (Boston Scientific Inc.) were used to generate personalized atrial models with annotation of anatomical regions. To reproduce patient-specific ERP, the established Courtemanche cellular model was gradually reparameterized from control conditions to a setup representing AF-induced remodeling. Three different approaches were studied for each patient’s digital twin: 1) a control scenario with no ERP personalization, in which the whole atrium had the same ERP, 2) a discrete split where each of the anatomical regions had a single ERP value and 3) a continuous ERP distribution by interpolation of measured data points over the entire atrial surface (Fig. 1). Arrhythmia vulnerability was subsequently assessed by virtual S1S2 pacing from different locations separated by 3 cm, yielding a total of 15 points for patient A and 12 for patient B. Induced tachycardias were classified as AF if the tachycardia cycle length (TCL) was less than 180ms and if electrical wavefronts collided at multiple locations. The number and location of inducing points and the resulting arrhythmia were determined for the control and for each personalization approach. The mean conduction velocity was set to 0.7 m/s and the electrical propagation in the atria was modeled by the monodomain equation and solved with openCARP.
Results
Clinical ERP measurements ranged from 200 to 270ms. Incorporating patient-specific ERP as a continuous ERP distribution did not induce any reentrant activity (Fig. 1). The mean induced TCL in silico for the regional scenario was 156±63ms for patient A and 122±9ms for patient B. For patient A, AF was induced at 3 different points with the control setup, whereas 9 ATs were induced with the regional method, of which 4 were AF and 5 macro reentries. For patient B, AF was induced at 1 point with the control setup; whereas with the regional approach, AF was induced at 4 points.
Conclusion
The results of this initial study show that the incorporation of patient-specific ERP values has an impact on the assessment of AF vulnerability. Furthermore, the type of personalization (regional vs. continuous) affects the likelihood of AF inducibility. The incorporation of more detailed ERP distributions may lead to a more accurate prediction of AF trigger points and could in the future inform patient-specific therapy planning. Larger cohorts need to follow to demonstrate the role of incorporating clinical patient-specific ERP values into personalized models for predicting AF vulnerability.
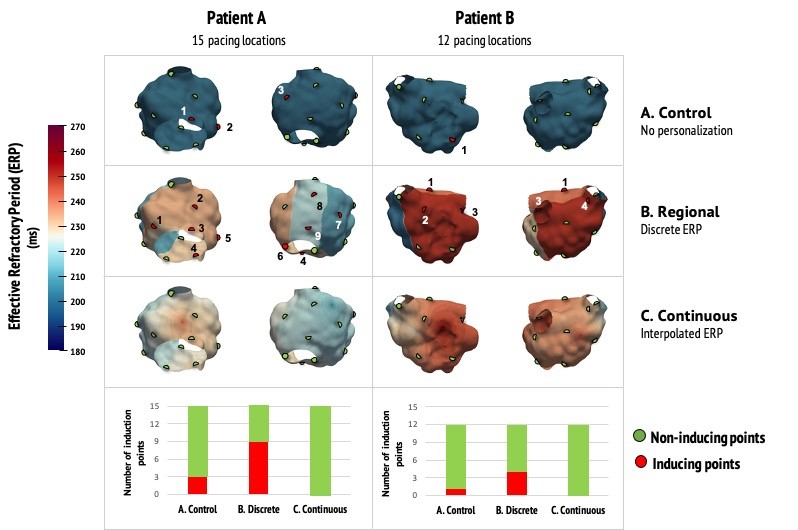
|
https://dgk.org/kongress_programme/jt2023/aP865.html
|