Background:
Heart failure is defined as the inability of the heart to meet body oxygen demand at rest or exercise, or to require an elevation in cardiac filling pressures in order to do this. Elevated left ventricular (LV) filling pressures play therefore a central role in this definition, and can be readily assessed in the cardiac catheterization laboratory. However, cardiac catheterization is invasive and time-consuming, so that the physicians, beside clinical examination, rather rely on non-invasive assessment of cardiac function based on ECG, pulse oximetry, ultrasound imaging, and biomarkers. A non-invasive and quick estimation of the left ventricle filling pressure would simplify and advance patients care.
Here, we set out to develop a novel machine-learning approach in order to predict the following, clinically relevant, LV indices: LV end-diastolic (Ped) and end-systolic pressure (Pes), as well as maximum rate of LV pressure rise (dPdt max).
Methods:
Synchronized invasive (LV pressure-volume signals) and non-invasive signals (ECG, pulse oximeter and phonocardiography via cardiac microphone) were collected from anesthetized, closed-chest instrumented Göttingen minipigs via a commercially available software (LabChart 10, Adinstruments). Animals were either i) healthy or had ii) a heart failure with reduced ejection fraction induced via right ventricular tachypacing over 6 weeks, ensuring a broad spectrum of LV Peds. A Matlab environment was used to extract a set of features from cardiac sounds, ECG, and pulse oximetry signals in order to predict the abovementioned LV indices via linear regression analysis. A total of ca. 500 heart cycles were included in the analysis.
Results:
The developed model was able to accurately predict the invasively measured LV Ped (figure below) with a correlation coefficient r2 of 0.955 and a RMSE (root mean square error) of 0.3 mmHg, i.e. a relative error of less than 2% in the indirect estimation of LV Ped. Similar results were obtained for LV Pes and dPdt max (r2 of 0.943 and 0.933, respectively).
Conclusions:
The newly developed machine-learning based algorithm allows for excellent prediction of LV pressure indices from simultaneously collected ECG, pulse oximetry and heart sounds. This algorithm could significantly advance diagnostics and care of heart failure patients.
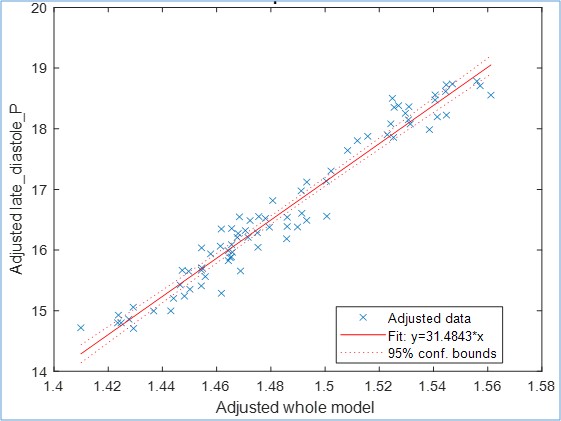