Clin Res Cardiol (2022). https://doi.org/10.1007/s00392-022-02002-5
|
Utility of Circulating Biomarkers for Robust Risk Prediction of Incident Atrial Fibrillation - Insights from the BiomarCaRE Project
|
B. Toprak1, S. Brandt1, J. Brederecke1, F. Gianfagna2, J. K. Vishram-Nielsen3, F. M. Ojeda1, S. Costanzo4, C. S. Börschel1, S. Söderberg5, I. Katsoularis5, S. Camen1, E. Vartiainen6, M. B. Donati4, J. Kontto6, M. Bobak7, E. B. Mathiesen8, A. Linneberg3, W. Koenig9, M.-L. Løchen10, A. Di Castelnuovo11, S. Blankenberg1, G. de Gaetano4, K. Kuulasmaa6, V. Salomaa6, L. Iacoviello2, T. Niiranen6, T. Zeller1, R. Schnabel1, für die Studiengruppen: BiomarCaRE, AFFECT-EU
|
1Klinik für Kardiologie, Universitäres Herz- und Gefäßzentrum Hamburg GmbH, Hamburg; 2Research Center in Epidemiology and Preventive Medicine (EPIMED), Department of Medicine and Surgery, University of Insubria, Varese, IT; 3Center for Clinical Research and Prevention, Bispebjerg and Frederiksberg Hospital, Frederiksberg, DK; 4Department of Epidemiology and Prevention, IRCCS Neuromed, Pozzilli, IT; 5Department of Public Health and Clinical Medicine, and Heart Centre, Umeå University, Umeå, SE; 6Department of Public Health and Welfare, Finnish Institute for Health and Welfare, Helsinki, FI; 7Department of Epidemiology and Public Health, University College London, London, UK; 8Brain and Circulation Research Group, Department of Clinical Medicine, UiT The Arctic University of Norway, Tromsø, NO; 9Klinik für Herz- und Kreislauferkrankungen, Deutsches Herzzentrum München, München; 10Department of Community Medicine, UiT The Arctic University of Norway, Tromsø, NO; 11Mediterranea Cardiocentro, Napoli, IT;
|
Introduction: Atrial fibrillation remains a major health issue in Europe and worldwide. Risk prediction is crucial to identify at-risk individuals and prevent subsequent complications of atrial fibrillation such as stroke and heart failure. Besides clinical risk factors, the use of circulating biomarkers mirroring different pathophysiological pathways of atrial fibrillation may potentially improve risk prediction in the general population.
Methods: Fourteen different biomarkers were measured in 42,280 individuals from three prospective European community cohorts (median age 52.2 years, 48.3% men). All individuals were free of atrial fibrillation and/or atrial flutter at baseline. First, we used multivariable-adjusted Cox regressions to investigate the association of each biomarker with incident atrial fibrillation, followed by analyses on discrimination and reclassification using 10-fold cross-validation for all single-marker models, a dual-marker model including high-sensitivity troponin I (hsTnI) and N-terminal pro B-type natriuretic peptide (NT-proBNP), and a multi-marker model with all investigated biomarkers. A base model incorporating clinical risk indicators was used as the reference. Second, machine learning methods, including Least Absolute Shrinkage and Selection Operator (LASSO) and Random Survival Forest (RSF) were applied to rank clinical variables and circulating biomarkers according to their importance for the prediction of incident atrial fibrillation.
Results: During a median follow-up of 5.7 years, 1,496 individuals (3.5%) were diagnosed with incident atrial fibrillation. In multivariable-adjusted regression analysis, NT-proBNP was the strongest circulating predictor of new-onset atrial fibrillation (hazard ratio [HR] per standard deviation [SD] 1.93, 95% confidence interval [CI] 1.82-2.04, P<0.001). In addition, hsTnI (HR per SD 1.18, 95% CI 1.13-1.22, P<0.001), cystatin C (HR per SD 1.16, 95% CI 1.10-1.23, P<0.001), CRP (HR per SD 1.08, 95% CI 1.02-1.14, P=0.012), total cholesterol (HR per SD 0.90, 95% CI 0.85-0.95, P<0.001), LDL cholesterol (HR per SD 0.93, 95% CI 0.88-0.98, P=0.007), apolipoprotein B (HR per SD 0.92, 95% CI 0.88-0.98, P=0.005), and triglycerides (HR per SD 0.92, 95% CI 0.87-0.98, P=0.009) were significantly related to incident atrial fibrillation. NT-proBNP was the only biomarker which improved discrimination (ΔC-index 0.037, 95% CI 0.029-0.044) and reclassification (net reclassification index [NRI] 0.237, 95% CI 0.187-0.287) markedly when compared to the clinical model (C-index 0.823, 95% CI 0.775-0.871). Neither the dual-marker model incorporating hsTnI in addition to NT-proBNP, nor a multi-marker model with all investigated biomarkers enhanced the predictive ability of NT-proBNP alone substantially. In different machine learning models, NT-proBNP and age remained the strongest predictors of incident atrial fibrillation.
Conclusions: Using a dual-track approach with classical statistical methods and modern machine learning techniques, NT-proBNP consistently remained the strongest circulating predictor of new-onset atrial fibrillation with outstanding discriminative capacity and reclassification yield. NT-proBNP-enriched, targeted screening in the general population may thus optimize the prediction of atrial fibrillation and potentially prevent related complications in real-world settings.
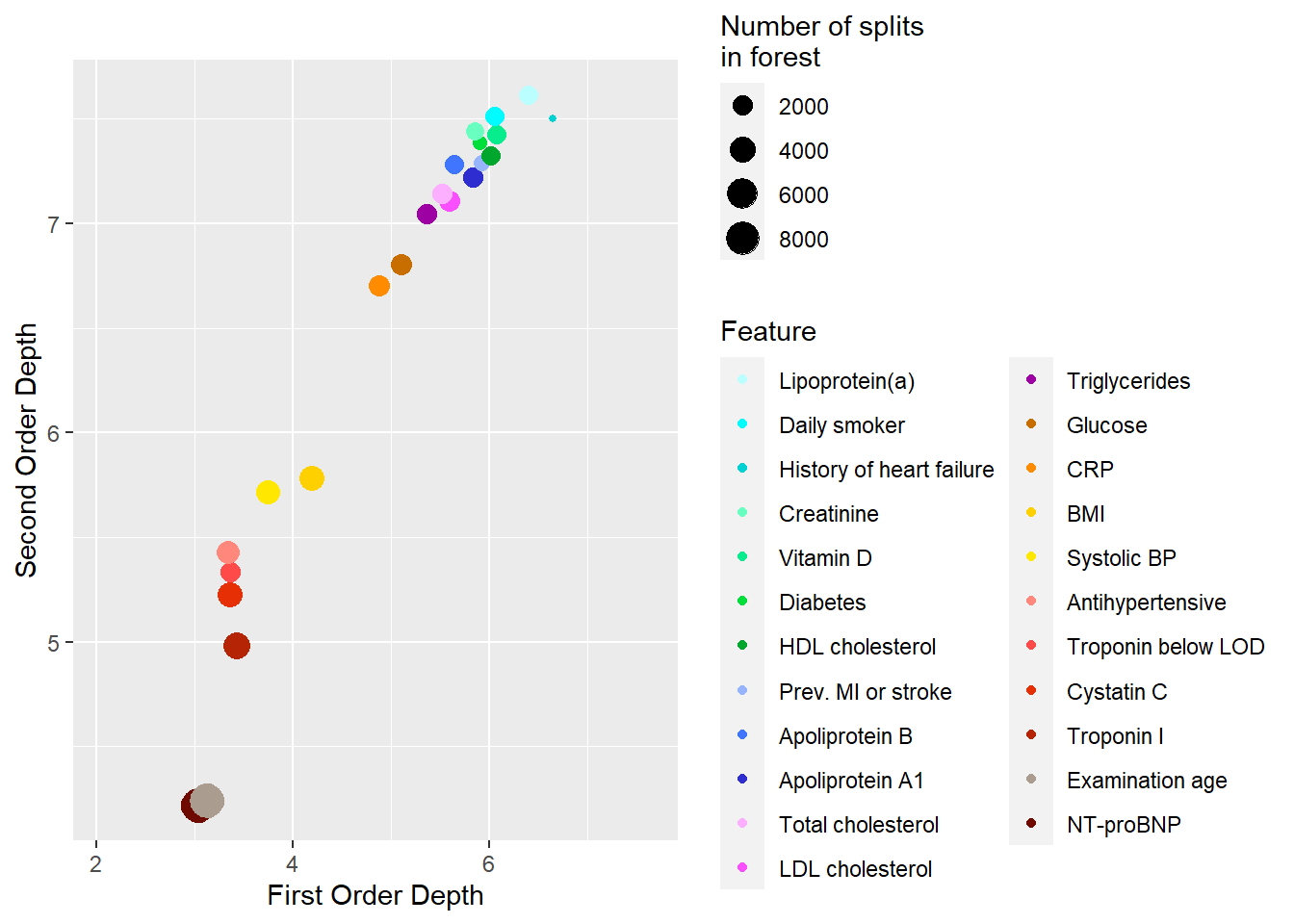
|
https://dgk.org/kongress_programme/jt2022/aV1275.html
|