Background:
Risk prediction in patients with chronic heart failure is essential to optimally tailor diagnostic and therapeutic strategies, and effectively use health care resources. Risk scores derived from clinical studies can be used to estimate the risk of mortality and heart failure hospitalizations for individual patients. However, these scores are poorly implemented into routine care, predominantly because their calculation requires considerable efforts in practice.
Objectives:
We selected the MAGGIC (M; 5 continuous and 8 categorical variables) and the Barcelona BioHF V1 (B; 5 continuous and 6 categorical variables) Heart Failure (HF) Scores to demonstrate a multi-site solution for score derivation based on data documented in routine clinical care (Table 1). Herein, we employ interoperability solution, which were established within HiGHmed one of the consortia of the Medical Informatics Initiative.
Methods:
Within HiGHmed, Medical Data Integration Centers (MeDICs) were established at 7 university hospitals. MeDICs integrate and share medical data collected during clinical routine, thereby making these data sets accessible and reusable for translational research and, ultimately, improved patient care. Our Use Case Cardiology (UCC) team established a multicenter registry of patients with chronic heart failure. We implemented a UCC harmonized data set (CDS), which based on DZHK guidelines and comprises various clinical domains, within the openEHR framework (selected variables are presented in Table 1). openEHR is a technology for e-health consisting of open platform specifications, clinical models and software, which enable data integration across all participating university hospitals. Our approach minimizes the need for manual data entry by automatically retrieving data from primary systems wherever possible. All MeDICs host local openEHR databases, which are used to obtain all required data items for the actual HF risk score calculation. We use the same programmatic queries across all partner sites and implemented our HF risk score calculation workflow in a common software package (written in R).
Results:
All 5 participating partner sites successfully executed data retrieval queries, followed by subsequent data processing and score calculation steps. From 2900 enrolled patients, we could compute M scores for 697 (24% of all patients) and B scores for 1550 (53%). Specific numbers for each study site are shown in Figure 1. Mean (SD) score was 0.157(0.116) for M and 0.092(0.098) for B, thus covering a large range of risk. We could identify poor documentation of selected items in clinical routine as one main reason for HiGHmed patients with missing HF risk scores. The most frequently missing information was “time of first heart failure diagnosis”.
Conclusions:
We show that an interoperable openEHR data set from clinical routine enabled easy and automated heart failure risk score calculation. We demonstrate workflow by an assessment of individual patients in a non-selected population of hospitalized patients with chronic heart failure. Our study serves as a first indicator that the use of standardized data models and terminologies has great potential for cross-site data analyses, which will open the door for reducing hospitalizations and mortality of high-risk HI patients through clinical decision support. The prognostic and clinical utility of this approach needs to be shown in prospective analyses.
Table 1
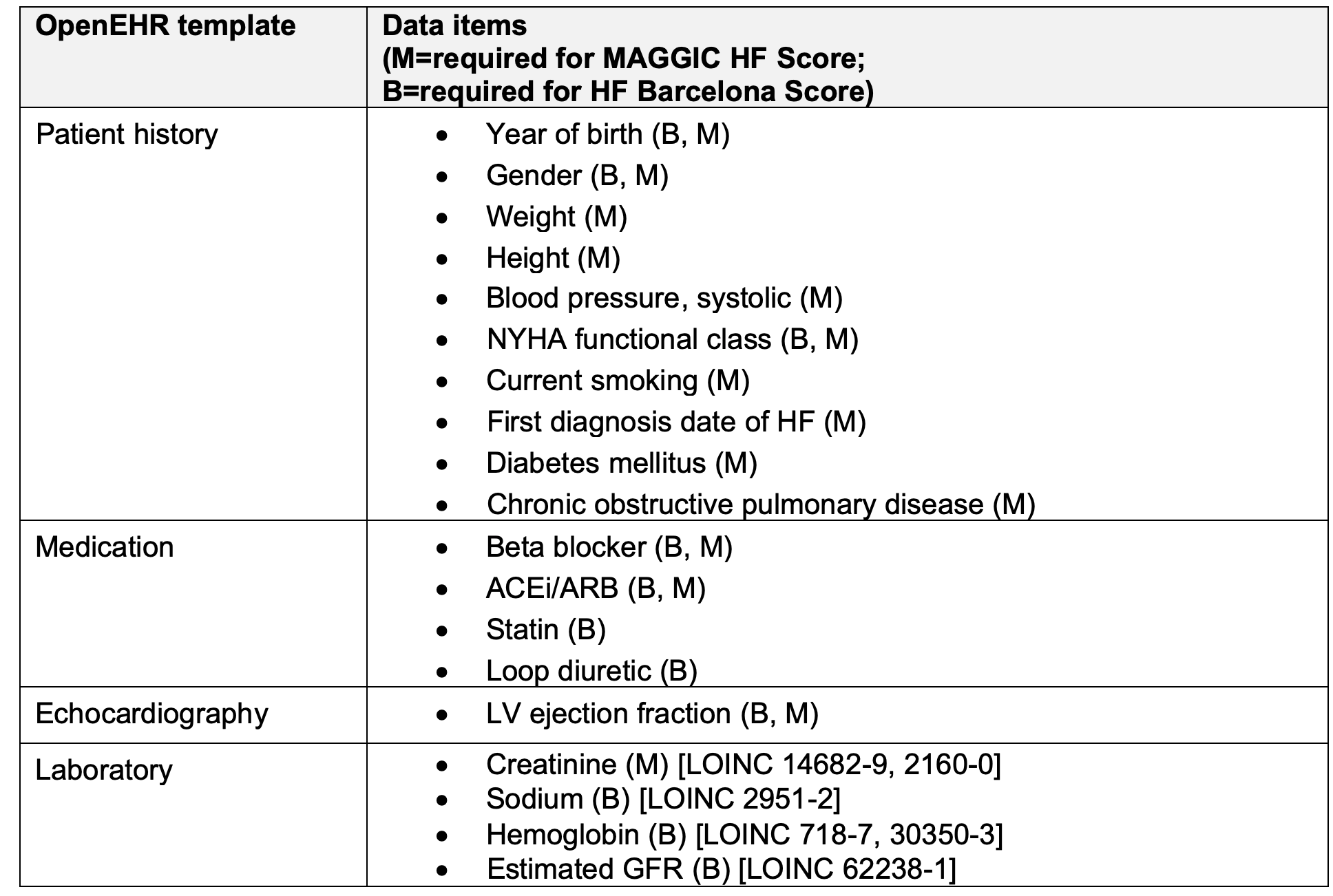
Figure 1
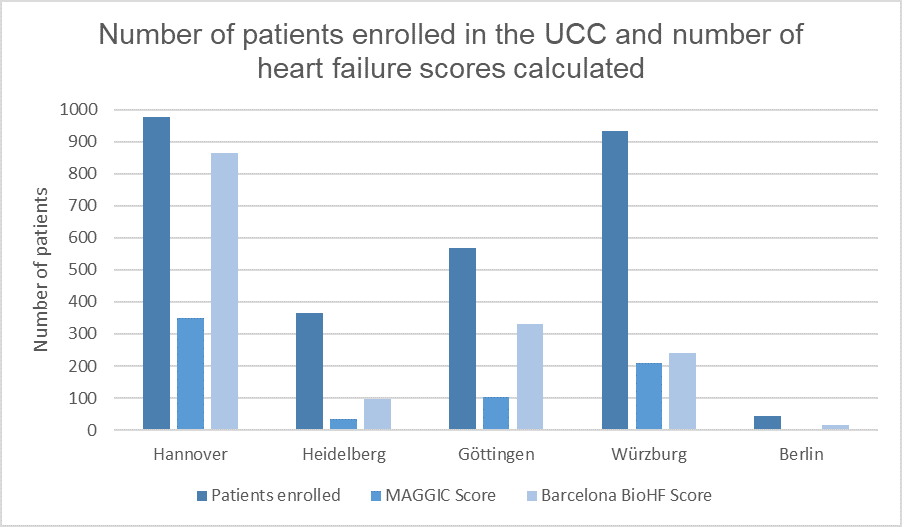