1Klinik für Innere Med. III, Kardiologie, Angiologie u. Pneumologie, Universitätsklinikum Heidelberg, Heidelberg; 2UT Southwestern Medical Center, Dallas, Texas, US; 3Cleveland Clinic, Cleveland, OH, US;
|
Background: Duchenne muscular dystrophy (DMD) is the most commonly inherited muscular disorder in childhood, where a fibrofatty replacement of myocardial segments causes cardiac failure. DMD follow-up examinations repeatedly require cardiac tissue characterisation through late gadolinium enhancement (LGE), a cardiac magnetic resonance imaging (CMR) technique where administered contrast agent accumulates in akinetic myocardial segments. The clinical best practice (CBP) to decide for LGE scans involves a cardiologist who utilises clinical software to visually inspect and evaluate the myocardial deformation in gadolinium-free cine-SSFP (native) CMR images. We hypothesise, that the automatic identification of akinetic myocardial segments in the native images decreases consecutive LGE examinations and reduces contrast administration in DMD follow-up. Our proposed novel fully automatic approach exploits the temporal information and utilises strain values in relation to five cardiac phases to predict the akinetic segments in the native images. In the CBP, only deformation in relation to end-diastole is considered.
Methods: The study involved 57 male DMD patients (mean age 14.1±3.2) who underwent CMR with LGE. We performed two steps on the native images of the mid-cavity region: First, an extraction of the myocardial motion between distinctive cardiac phases with deep learning, second, a classification of the myocardial segments into akinetic/healthy with a support vector classifier (SVC). In the first step, a deformable volume registration returned five dense vector fields, which described grey values’ motion between five distinctive cardiac phases: ED to mid-systole (MS), MS to ES, ES to peak-flow (PF), PF to mid-diastole (MD), MD to ED. Each vector field was spatially divided into six segments (American Heart Association, AHA) and radial/circumferential strain (RS, CS) maps were calculated. The resulting temporal strain maps per segment were fed into a SVC to predict akinetic myocardial segments. The cardiac segments with akinetic motion predicted through a) the semi-manual CBP and b) our novel fully automatic model were compared to the LGE ground truth images, where negative and positive myocardial contrast uptakes indicate healthy (LGE-) and akinetic myocardium (LGE+), respectively (Fig.1).
Results: Statistical analysis was performed using unequal variances t-test. The mean mid-cavity RS/CS peak values were 49.65±19.02, -2.32±3.02 in the LGE- and 41.87±22.36, -1.65±2.49 in the LGE+ patients (p<0.001, p<0.03). Highest absolute strain differences between LGE-/LGE+ were observed at the following segments and phase transitions: AHA7,9 at ED-MS; AHA10,11,12 at MS-ES; AHA8 at ES-PF (RS) and AHA8 at ED-MS; AHA7,11,12 at ES-PF; AHA9 at PF-MD; AHA10 at MD-ED (CS) (Fig.2). The semi-manual classification in CBP achieved a balanced accuracy, precision, recall and F1-score of 0.72, 0.74, 0.81 and 0.78, with 26 true positive (TP), 15 true negative (TN), 9 false positive (FP), 6 false negative (FN) cases. The Area Under the Curve (AUC) was 0.72. In comparison, our approach reached 0.88±0.10, 0.88±0.15, 0.93±0.13 and 0.89±0.09, with 26 TP, 23 TN, 5 FP, 2 FN cases and an AUC of 0.88 (+16%).
Conclusion: Our novel fully automatic approach outperforms the CBP by +16% accuracy and +12% recall through strain analysis at five distinctive cardiac phases. This promising approach may reduce the necessity of gadolinium-based contrast administration in DMD follow-up.
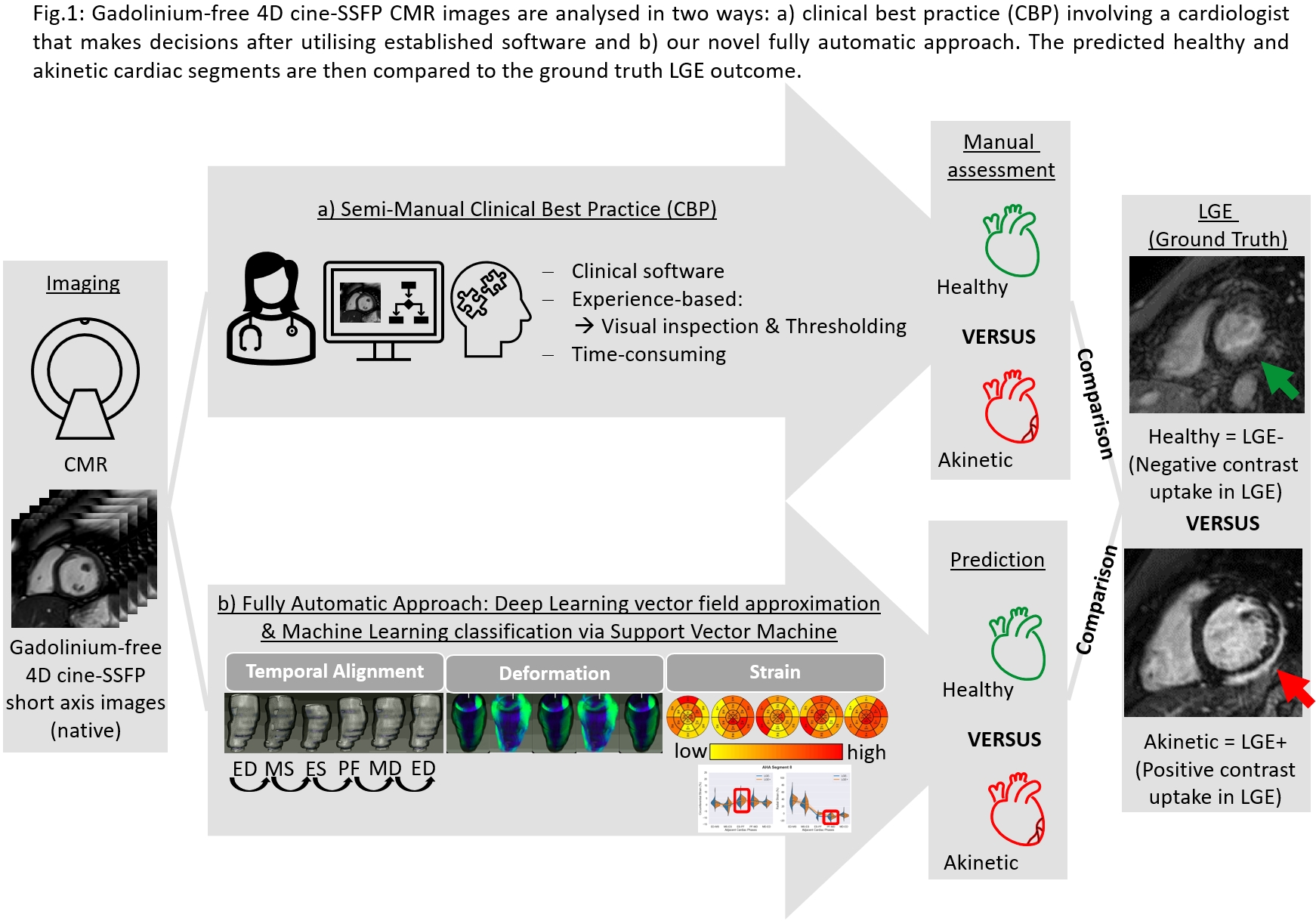
|